Centralized Data Warehouse vs. Data Mesh: Transforming Data Management
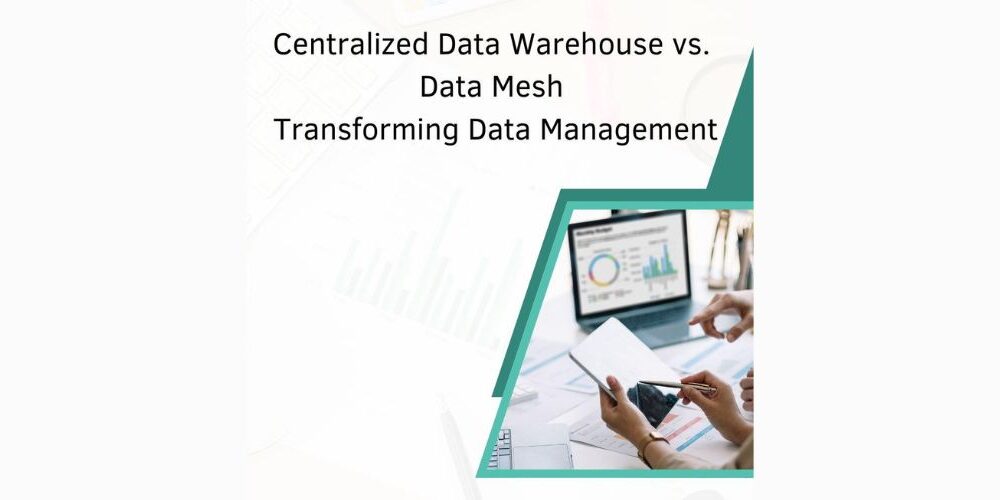
In the dynamic realm of data management, Santhosh Gourishetti brings forward a compelling comparative analysis of two significant paradigms: centralized data warehouses and data mesh architectures. This article explores the innovative shifts these paradigms bring to modern enterprises striving to harness data’s potential.
The Evolution of Data Management
Data volumes are growing unprecedentedly, projected to reach 180 zettabytes by 2025. Managing this explosive growth has driven organizations to reassess their strategies, giving rise to transformative models like centralized data warehouses and data meshes. While distinct in principles and execution, these approaches share a common goal—empowering businesses with scalable, reliable, and actionable insights.
Centralized Data Warehouses: The Established Pillar
Unified Data Integration
Centralized data warehouses have long been the backbone of enterprise data strategies. By consolidating data from various sources into a unified repository, they ensure consistency and offer a single authoritative source of truth. This integration simplifies analytics and decision-making, fostering confidence in the insights derived.
Governance and Compliance
A hallmark of centralized systems is their robust governance. They enable organizations to implement standardized policies, ensuring data security and regulatory compliance. Such centralization minimizes discrepancies and enforces uniformity across the enterprise.
Challenges of Scalability
Despite their advantages, centralized warehouses struggle with scalability. The exponential growth of data imposes significant infrastructure demands, leading to potential bottlenecks. Moreover, their rigid schemas can hinder adaptability to rapidly changing business requirements.
Data Mesh: A Paradigm Shift
Decentralized Ownership
Data mesh introduces a domain-oriented approach, decentralizing data management across specialized teams. This shift empowers teams to treat data as a product, tailoring it to specific needs while fostering ownership and accountability.
Agility and Scalability
This architecture’s decentralized nature offers unmatched scalability and agility. By enabling independent evolution of domain-specific data products, data mesh facilitates rapid adaptation to business changes without disrupting the broader system.
Governance Complexities
However, data mesh comes with its challenges. Ensuring consistent governance across decentralized domains requires robust frameworks. Integrating data for cross-functional insights can also be complex, necessitating advanced coordination mechanisms.
Comparative Insights
Control vs. Flexibility
Centralized data warehouses excel in organizational control, offering strong governance and consistency. In contrast, data mesh prioritizes flexibility and scalability, accommodating diverse and evolving needs at the expense of centralized oversight.
Adaptability to Business Needs
For businesses requiring swift adaptations, data mesh proves superior. Its domain-driven design enables teams to adjust quickly to market dynamics. Centralized warehouses, while stable, often lag in accommodating rapid changes.
Data Quality and Integration
Centralized systems’ unified architecture simplifies data quality management but may face performance limitations with large-scale operations. Data mesh’s domain-specific focus can enhance quality within domains but risks creating silos without effective integration strategies.
The Rise of Hybrid Models
Organizations increasingly explore hybrid approaches to balance the strengths of both paradigms. A core-and-spoke model, for instance, combines centralized governance with decentralized execution. Federated data virtualization offers another solution, providing a centralized view of distributed data sources.
Strategic Considerations
Choosing between centralized and decentralized architectures depends on organizational needs. Centralized warehouses suit smaller setups with simpler requirements, while larger enterprises handling diverse data may benefit from data meshes or hybrid models. Additionally, industry regulations and legacy infrastructures significantly influence the selection and implementation of data management strategies.
Innovating for the Future
The insights illuminate the intricate dynamics between centralized systems, data mesh architectures, and hybrid models, encouraging organizations to evaluate their unique needs and operational contexts. By leveraging the stability of centralized systems, the agility of a data mesh, or the balance provided by hybrid approaches, organizations can pave the way for innovation. The ultimate goal is to create a resilient, scalable, and adaptive data management framework that fosters sustainable growth and long-term success.
In conclusion, Santhosh Gourishetti emphasizes that embracing innovative data management strategies is crucial for organizational success in today’s data-driven era. By adapting to evolving demands and leveraging advanced analytics, businesses can uncover new opportunities and achieve long-term growth. Gourishetti’s insightful analysis highlights the transformative impact of effective data management. It is a practical guide for leaders to navigate this complex landscape, ensuring their organizations remain competitive and resilient in an ever-changing digital world.